The study on the predictive accuracy of artificial intelligence (AI) Lunit INSIGHT CXR Version 3.0 for pneumonia diagnosis in COVID-19 patients
DOI:
https://doi.org/10.46475/asean-jr.v24i3.881Keywords:
Artificial intelligence, Chest radiograph, Covid-19, Diagnosis, PneumoniaAbstract
Background: Millions of people in Thailand have been infected and died from the infection of the COVID-19. As a result, the country’s public health system is greatly affected due to the limitation of the number of physicians. Artificial intelligence (AI) is, therefore, used to reduce the working load of physicians in the diagnosis of COVID-19 patients.
Objective: To study on the predictive accuracy of AI Lunit INSIGHT CXR Version 3.0 for pneumonia diagnosis in COVID-19 patients.
Materials and Methods: This study was a retrospective study. The data was collected from 256 confirmed cases of COVID-19 infection admitted as new patients in the Nimibutr Pre-Admission Centre of the Institute of Neurology, the Ministry of Public Health. They were randomly selected from the database.
Seven radiologists and Lunit INSIGHT CXR Version 3.0 software interpret the CXR film to diagnose pneumonia in COVID-19 patients from chest radiographs (CXR).
Results: The research results of the diagnosis of pneumonia in patients infected with COVID-19 between from radiologists and using AI Lunit INSIGHT CXR Version 3.0 software revealed 97.87% (95%CI 88.71-99.95%) of sensitivity, 99.04% (95%CI 96.59-99.88%) of specificity, accuracy = 98.83%, positive predictive value (PPV) = 95.83%, and negative predictive value (NPV) = 99.52%, positive likelihood ratio (+LR) = 102.28, negative likelihood ratio (-LR) = 0.02.
Conclusion: The artificial intelligence software Lunit INSIGHT CXR Version 3.0 can be used to interpret the diagnosis of pneumonia in patients infected with COVID-19 in order to reduce radiologists’ workloads during the COVID pandemic when medical staff were limited.
Downloads
Metrics
References
who.int [Internet]. Geneva: WHO; c2023 [cited 2023 Dec 27]. Coronavirus disease (COVID-19) pandemic. Available from: https://www.who.int/emergencies/diseases/novel-coronavirus-2019
Thanachotiphan K, Kalyanamitra K, Niyomyaht S, Kakkhanpichonchat T. [Management of Thai Health Service Systems During the Covid-19 Crisis]. J L G ISRRU 2022; 6(2):111-29. Thai.
Department of Physical Education, Ministry of Tourism and Sports [Internet]. 2021 [cited 2023 Dec 27]. Opening the Nimibutr Pre-Admission Center ready to support those infected with COVID-19. Available from: https://dpe.go.th/news-preview-431191791858. Thai.
Xie X, Zhong Z, Zhao W, Zheng C, Wang F, Liu J. Chest CT for typical coronavirus disease 2019 (COVID-19) pneumonia: relationship to negative RT-PCR testing. Radiology 2020;296:E41-5. doi: 10.1148/radiol.2020200343.
Suwatanapongched T, Nitiwarangkul C, Sukkaseam W, Phongkitkarun S. Rama Co-RADS: Categorical Assessment Scheme of Chest Radiographic Findings for Diagnosing Pneumonia in Patients with Confirmed COVID-19. Rama Med J 2022; 44(2):50-62.
Benza RL, Gomberg-Maitland M, Elliott CG, Farber HW, Foreman AJ, Frost AE, et al. Predicting survival in patients with pulmonary arterial hypertension: the REVEAL risk score calculator 2.0 and comparison with ESC/ERS-based risk assessment strategies. Chest 2019;156:323-37. doi: 10.1016/j.chest.2019.02.004.
Kwee TC, Kwee RM. Chest CT in COVID-19: what the radiologist needs to know. Radiographics 2020;40:1848-65. doi: 10.1148/rg.2020200159.
Larici AR, Cicchetti G, Marano R, Merlino B, Elia L, Calandriello L, et al. Multimodality imaging of COVID-19 pneumonia: from diagnosis to follow-up. A comprehensive review. Eur J Radiol 2020;131:109217. doi: 10.1016/j.ejrad.2020.109217.
Litmanovich DE, Chung M, Kirkbride RR, Kicska G, Kanne JP. Review of chest radiograph findings of COVID-19 pneumonia and suggested reporting language. J Thorac Imaging 2020;35:354-60. doi: 10.1097/RTI.0000000000000541.
Ardakani AA, Kanafi AR, Acharya UR, Khadem N, Mohammadi A. Application of deep learning technique to manage COVID-19 in routine clinical practice using CT images: Results of 10 convolutional neural networks. Comput Biol Med 2020;121:103795. doi: 10.1016/j.compbiomed.2020.103795.
Gatt ME, Spectre G, Paltiel O, Hiller N, Stalnikowicz R. Chest radiographs in the emergency department: is the radiologist really necessary?. Postgrad Med J 2003;79(930):214-7. doi: 10.1136/pmj.79.930.214.
Jacobi A, Chung M, Bernheim A, Eber C. Portable chest X-ray in coronavirus disease-19 (COVID-19): A pictorial review. Clin Imaging 2020;64:35–42. doi: 10.1016/j.clinimag.2020.04.001.
Guo W, Wang J, Sheng M, Zhou M, Fang L. Radiological findings in 210 paediatric patients with viral pneumonia: a retrospective case study. Br J Radiol 2012;85:1385–9. doi: 10.1259/bjr/20276974.
Lomoro P, Verde F, Zerboni F, Simonetti I, Borghi C, Fachinetti C, et al. COVID-19 pneumonia manifestations at the admission on chest ultrasound, radiographs, and CT: single-center study and comprehensive radiologic literature review. Eur J Radiol Open 2020;7:100231. doi: 10.1016/j.ejro.2020.100231.
Baltazar LR, Manzanillo MG, Gaudillo J, Viray ED, Domingo M, Tiangco B, et al. Artificial intelligence on COVID-19 pneumonia detection using chest xray images. PloS One 2021;16:e0257884. doi: 10.1371/journal.pone.0257884.
Hosny A, Parmar C, Quackenbush J, Schwartz LH, Aerts HJWL. Artificial intelligence in radiology. Nat Rev Cancer 2018;18:500-10. doi: 10.1038/s41568-018-0016-5.
Syed AB, Zoga AC. Artificial intelligence in radiology: current technology and future directions. Semin Musculoskelet Radiol 2018;22: 540-5. doi: 10.1055/s-0038-1673383.
Ávila-Tomás JF, Mayer-Pujadas MA, Quesada-Varela VJ. [Artificial intelligence and its applications in medicine II: current importance and practical applications]. Aten Primaria 2020;53:81-8. Spanish. doi: 10.1016/j.aprim.2020.04.014.
Mahatchariyapong P. Evaluation of the diagnostic accuracy of Artigivisl Intelligence detection of pulmonary tuberculosis on Chest Radiograph among outpatients in Maeramard district, Thailand. J Prim Care Fam Med 2022; 4:35-45.
Borkowski AA, Viswanadhan NA, Thomas LB, Guzman RD, Deland LA, Mastorides SM. Using artificial intelligence for COVID-19 chest X-ray diagnosis. Fed Pract 2020;37:398-404. doi: 10.12788/fp.0045.
Faul F, Erdfelder E, Lang AG, Buchner A. G* Power 3: A flexible statistical power analysis program for the social, behavioral, and biomedical sciences. Behav Res Methods. 2007;39:175-91. doi: 10.3758/bf03193146.
Shin HJ, Han K, Ryu L, Kim EK. The impact of artificial intelligence on the reading times of radiologists for chest radiographs. NPJ Digit Med 2023;6:82. doi: 10.1038/s41746-023-00829-4.
Becker J, Decker JA, Römmele C, Kahn M, Messmann H, Wehler M, Schwarz F, Kroencke T, Scheurig-Muenkler C. Artificial intelligence-based detection of pneumonia in chest radiographs. Diagnostics (Basel) 2022;12:1465. doi: 10.3390/diagnostics12061465.
Vasilev Y, Vladzymyrskyy A, Omelyanskaya O, Blokhin I, Kirpichev Y, Arzamasov K. AI-Based CXR First Reading: Current Limitations to Ensure Practical Value. Diagnostics (Basel) 2023;13:1430. doi: 10.3390/diagnostics13081430.
Mei X, Lee HC, Diao KY, Huang M, Lin B, Liu C, et al. Artificial intelligence-enabled rapid diagnosis of patients with COVID-19. Nat Med 2020;26:1224–8. doi: 10.1038/s41591-020-0931-3.
Khanna VV, Chadaga K, Sampathila N, Prabhu S, Chadaga R, Umakanth S. Diagnosing COVID-19 using artificial intelligence: A comprehensive review. Network Modeling Analysis in Health Informatics and Bioinformatics 2022;11(1):25.
Harmon SA, Sanford TH, Xu S, Turkbey EB, Roth H, Xu Z, et al.Artificial intelligence for the detection of COVID-19 pneumonia on chest CT using multinational datasets. Nature communications. 2020 Aug 14;11(1):4080. doi: 10.1038/s41467-020-17971-2.
Noisiri W, Vijitrsaguan C, Lertrojpanya S, Jiamjit K, Chayjaroon J, Tantibundhit C. Sensitivity and Specificity of Artificial Intelligence for Chest Diagnostic Radiology in Lung Cancer. J Depart Med Ser 2021;45(4):55-61.
Dorr F, Chaves H, Serra MM, Ramirez A, Costa ME, Seia J, et al. COVID-19 pneumonia accurately detected on chest radiographs with artificial intelligence. Intell Based Med 2020;3:100014. doi: 10.1016/j.ibmed.2020.100014.
Alexander R, Waite S, Bruno MA, Krupinski EA, Berlin L, Macknik S, et al.Mandating limits on workload, duty, and speed in radiology. Radiology 2022 ;304:274-82. doi: 10.1148/radiol.212631.
Taylor-Phillips S, Stinton C. Fatigue in radiology: a fertile area for future research. Br J Radiol 2019;92:20190043. doi: 10.1259/bjr.20190043.
Van Beek EJR, Ahn JS, Kim MJ, Murchison JT. Validation study of machine-learning chest radiograph software in primary and emergency medicine. Clin Radiol 2023 ;78:1-7. doi: 10.1016/j.crad.2022.08.129.
Trimankha P, Lakkana Jirapong L, Rungsin R, Autravisittikul O, Deesuwan PDM, Mekavuthikul Y, et al. Utility of screening chest radiographs in patients with asymptomatic and mildly symptomatic COVID-19 at a field hospital in Samut Sakhon, Thailand. ASEAN J Radiol [Internet]. 2021[cited 2023 Dec 28];22(2):05-20. Available from: https://www.asean-journal-radiology.org/index.php/ajr/article/view/119.
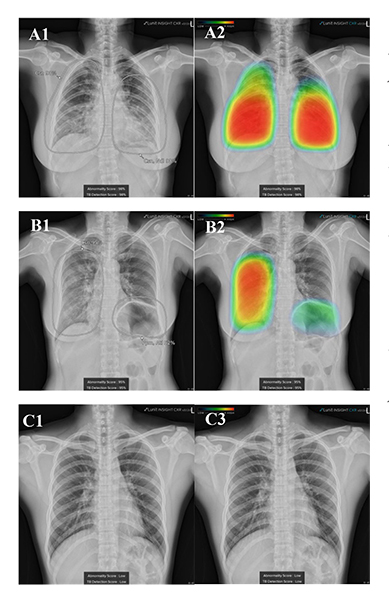
Downloads
Published
How to Cite
Issue
Section
License
Copyright (c) 2023 The ASEAN Journal of Radiology

This work is licensed under a Creative Commons Attribution-NonCommercial-NoDerivatives 4.0 International License.
Disclosure Forms and Copyright Agreements
All authors listed on the manuscript must complete both the electronic copyright agreement. (in the case of acceptance)