Reliability and radiologists’ concordance of artificial intelligence (AI)-calculated Alberta Stroke Program Early CT Score (ASPECTS)
DOI:
https://doi.org/10.46475/asean-jr.v25i3.901Keywords:
Acute ischemic stroke, Alberta Stroke Program Early CT Score, Artificial intelligence, Computed tomographyAbstract
Abstract
Background: ASPECTS was developed for the semi-quantitative assessment of early ischemic changes (EIC) on non-contrast computed tomography (NCCT) in acute ischemic stroke (AIS). Artificial intelligence (AI)-based automated tools for the ASPECT scoring system were developed to automate the diagnosis and improve the agreement with radiologists of AIS. The performance of the automated software compared to physicians should be tested before the software is further used in clinical practice as a tool for clinicians.
Objective: To evaluate the agreement with radiologists of an AI-based automated post-processing software for detecting EIC and calculating ASPECTS on NCCT images in AIS patients using a radiologist's assessment as a reference.
Materials and Methods: NCCT of AIS patients were retrospectively reviewed (Stroke Fast Track Service July 2022 - December 2023). The complete set of clinical data and imaging data from both baseline and follow-up were analyzed by a radiologist as a reference. Two additional observers provided individual ASPECTS from the baseline NCCT only (observer 1 was a radiologist who independently reviewed only the baseline NCCT with stroke window setting. Observer 2 was a radiologist on service which was from the pool of 20 radiologists onsite and online). Recon&GO Inline ASPECTS software (Somaris X, VA40A, Siemens Healthineers AG, Erlangen, Germany) was applied. Both ASPECT score analysis and ASPECTS region analysis were evaluated. Positive percent agreement (PPA) and negative percent agreement (NPA) were calculated. Interobserver agreement was assessed using the Cohen's kappa coefficient and the intraclass correlation coefficient (ICC).
Results: 111 patients with a mean age of 67.8 years (±11.9), 56 (50.5%) females, a mean National Institute of Health Stroke Scale (NIHSS) score of 14.2 (±8.8), and a mean time to baseline NCCT of 123.9 minutes (±58.7) were included. For dichotomized ASPECTS, the automated software showed lower PPA (14.6% vs. 27.1%) but higher NPA (100.0% vs. 93.7%) than observer 2. For the region-based analysis, both the automated software and observer 2 differed in terms of regional contribution. The automated software showed low PPA but rather high NPA with perfect (100%) NPA in lentiform nucleus and M2. The automated software showed higher agreement with the reference and two observers in deep/central regions than cortical regions. For total ASPECTS, the automated software showed a moderate agreement of total ASPECTS with the reference and observer 1 (ICC = 0.545 and 0.545). Observer 2 showed a poor agreement of total ASPECTS with the reference, observer 1, and the automated software (ICC = 0.349, 0.422, and 0.301, respectively).
Conclusion: For total ASPECT score, the agreement of the tested AI software is lower compared to observer 1 obtained by a radiologist using the stroke window on NCCT, but better compared to a pool of radiologists on service with a time limit of 30 minutes to interpret the ASPECT score. When analyzing the ASPECTS regions, there are different advantages for the assessment of the deep regions and the cortical regions. The tested AI software shows higher agreement in deep/central regions than cortical regions. From the result, the tested AI software retains its potential for use in emergency situations, particularly for radiologists with limited experience and limited time to report.
Downloads
Metrics
References
Soun JE, Chow DS, Nagamine M, Takhtawala RS, Filippi CG, Yu W, et al. Artificial intelligence and acute stroke Imaging. AJNR Am J Neuroradiol 2021;42:2-11. doi: 10.3174/ajnr.A6883.
Boehme AK, Esenwa C, Elkind MS. Stroke risk factors, genetics, and prevention. Circ Res 2017;120:472-95. doi: 10.1161/CIRCRESAHA.116.308398.
Emberson J, Lees KR, Lyden P, Blackwell L, Albers G, Bluhmki E, et al. Effect of treatment delay, age, and stroke severity on the effects of intravenous thrombolysis with alteplase for acute ischaemic stroke: a meta-analysis of individual patient data from randomised trials. Lancet 2014;384:1929-35. doi: 10.1016/S0140-6736(14)60584-5.
Tiamkao S. [13 years - learning and development of Stroke Fast Track in Thailand]. J Health Syst Res 2023;17:191-99. Thai.
Berkhemer OA, Fransen PS, Beumer D, van den Berg LA, Lingsma HF, Yoo AJ, et al. A randomized trial of intraarterial treatment for acute ischemic stroke. N Engl J Med 2015;372:11-20. doi: 10.1056/NEJMoa1411587.
Campbell BC, Mitchell PJ, Kleinig TJ, Dewey HM, Churilov L, Yassi N, et al. Endovascular therapy for ischemic stroke with perfusion-imaging selection. N Engl J Med 2015;372:1009-18. doi: 10.1056/NEJMoa1414792.
Albers GW, Marks MP, Kemp S, Christensen S, Tsai JP, Ortega-Gutierrez S, et al. Thrombectomy for stroke at 6 to 16 hours with selection by perfusion imaging. N Engl J Med 2018;378:708–18. doi: 10.1056/NEJMoa1713973.
Saver JL, Goyal M, Bonafe A, Diener HC, Levy EI, Pereira VM, et al. Stent-retriever thrombectomy after intravenous t-PA vs. t-PA alone in stroke. N Engl J Med 2015;372:2285-95. doi: 10.1056/NEJMoa1415061.
Goyal M, Demchuk AM, Menon BK, Eesa M, Rempel JL, Thornton J, et al. Randomized assessment of rapid endovascular treatment of ischemic stroke. N Engl J Med 2015;372:1019-30. doi: 10.1056/NEJMoa1414905.
Jovin TG, Chamorro A, Cobo E, de Miquel MA, Molina CA, Rovira A, et al. Thrombectomy within 8 hours after symptom onset in ischemic stroke. N Engl J Med 2015;372:2296-306. doi: 10.1056/NEJMoa1503780.
Nogueira RG, Jadhav AP, Haussen DC, Bonafe A, Budzik RF, Bhuva P, et al. Thrombectomy 6 to 24 hours after stroke with a mismatch between deficit and Infarct. N Engl J Med 2018;378:11-21. doi: 10.1056/NEJMoa1706442.
Turc G, Bhogal P, Fischer U, Khatri P, Lobotesis K, Mazighi M, et al. European Stroke Organisation (ESO) - European Society for Minimally Invasive Neurological Therapy (ESMINT) guidelines on mechanical thrombectomy in acute ischemic stroke. J Neurointerv Surg 2023;15:e8. doi: 10.1136/neurintsurg-2018-014569.
Powers WJ, Rabinstein AA, Ackerson T, Adeoye OM, Bambakidis NC, Becker K, et al. Guidelines for the early management of patients with acute ischemic stroke: 2019 update to the 2018 guidelines for the early management of acute ischemic stroke: a guideline for healthcare professionals from the American Heart Association/American Stroke Association. Stroke 2019;50:e344-e418. doi: 10.1161/STR.0000000000000211.
Venema E, Mulder MJHL, Roozenbeek B, Broderick JP, Yeatts SD, Khatri P, et al. Selection of patients for intra-arterial treatment for acute ischaemic stroke: development and validation of a clinical decision tool in two randomized trials. BMJ 2017;357:j1710. doi: 10.1136/bmj.j1710.
Barber PA, Demchuk AM, Zhang J, Buchan AM. Validity and reliability of a quantitative computed tomography score in predicting outcome of hyperacute stroke before thrombolytic therapy. ASPECTS Study Group. Alberta Stroke Programmed Early CT Score. Lancet 2000;355:1670-4. doi: 10.1016/s0140-6736(00)02237-6.
Pexman JH, Barber PA, Hill MD, Sevick RJ, Demchuk AM, Hudon ME, et al. Use of the Alberta Stroke Program Early CT Score (ASPECTS) for assessing CT scans in patients with acute stroke. AJNR Am J Neuroradiol 2001;22:1534-42.
Prakkamakul S, Yoo AJ. ASPECTS CT in acute ischemia: review of current data. Top Magn Reson Imaging 2017;26:103-12. doi: 10.1097/RMR.0000000000000122.
Herweh C, Ringleb PA, Rauch G, Gerry S, Behrens L, Möhlenbruch M, et al. Performance of e-ASPECTS software in comparison to that of stroke physicians on assessing CT scans of acute ischemic stroke patients. Int J Stroke 2016;11:438-45. doi: 10.1177/1747493016632244.
Lee EJ, Kim YH, Kim N, Kang DW. Deep into the brain: artificial intelligence in stroke imaging. J Stroke 2017;19:277-85. doi: 10.5853/jos.2017.02054.
Mokli Y, Pfaff J, Dos Santos DP, Herweh C, Nagel S. Computer-aided imaging analysis in acute ischemic stroke – background and clinical applications. Neurol Res Pract 2019;1:23. doi: 10.1186/s42466-019-0028-y.
Tang FH, Ng DK, Chow DH. An image feature approach for computer-aided detection of ischemic stroke. Comput Biol Med 2011;41:529-36. doi: 10.1016/j.compbiomed.2011.05.001.
Chen L, Bentley P, Rueckert D. Fully Automatic Acute Ischemic Lesion Segmentation in DWI Using Convolutional Neural
Networks. NeuroImage Clin 2017;15:633–43. doi: 10.1016/j.nicl.2017.06.016.
Kuang H, Najm M, Chakraborty D, Maraj N, Sohn SI, Goyal M, et al. Automated ASPECTS on noncontrast CT scans in patients with acute ischemic stroke using machine learning. AJNR Am J Neuroradiol 2019;40:33-8. doi: 10.3174/ajnr.A5889.
Takahashi N, Lee Y, Tsai DY, Matsuyama E, Kinoshita T, Ishii K. An automated detection method for the MCA dot sign of acute stroke in unenhanced CT. Radiol Phys Technol 2014;7:79-88. doi: 10.1007/s12194-013-0234-1.
Chen Y, Dhar R, Heitsch L, Ford A, Fernandez-Cadenas I, Carrera C, et al. Automated quantification of cerebral edema following hemispheric Infarction: Application of a machine-learning algorithm to evaluate CSF shifts on serial head CTs. NeuroImage Clin 2016;12:673–80. doi: 10.1016/j.nicl.2016.09.018.
Mainali S, Wahba M, Elijovich L. Detection of early ischemic changes in noncontrast CT head improved with "Stroke Windows". ISRN Neurosci 2014;2014:654980. doi: 10.1155/2014/654980.
Landis JR, Koch GG. The measurement of Observer agreement for categorical data. Biomentrics 1977; 33:159-74.
Koo TK, Li MY. A guideline of selecting and reporting intraclass correlation coefficients for reliability research. J Chiropr Med 2016;15:155-63. doi: 10.1016/j.jcm.2016.02.012.
Shieh Y, Chang CH, Shieh M, Lee TH, Chang YJ, Wong HF, et al. Computer-aided diagnosis of hyperacute stroke with thrombolysis decision support using a contralateral comparative method of CT image analysis. J Digit Imaging 2014 ;27:392-406. doi: 10.1007/s10278-013-9672-x.
Stoel BC, Marquering HA, Staring M, Beenen LF, Slump CH, Roos YB, et al. Automated brain computed tomographic densitometry of early ischemic changes in acute stroke. J Med Imaging (Bellingham) 2015;2:014004. doi: 10.1117/1.JMI.2.1.014004.
Ng YS, Stein J, Ning M, Black-Schaffer RM. Comparison of clinical characteristics and functional outcomes of ischemic stroke in different vascular territories. Stroke 2007;38:2309-14. doi: 10.1161/STROKEAHA.106.475483.
Mair G, Boyd EV, Chappell FM, von Kummer R, Lindley RI, Sandercock P, et al. Sensitivity and specificity of the hyperdense artery sign for arterial obstruction in acute ischemic stroke. Stroke 2015;46:102-7. doi:10.1161/STROKEAHA.114.007036.
Lim J, Magarik JA, Froehler MT. The CT-defined hyperdense arterial sign as a marker for acute intracerebral large vessel occlusion. J Neuroimaging 2018;28:212-6. doi: 10.1111/jon.12484.
Jensen-Kondering U, Riedel C, Jansen O. Hyperdense artery sign on computed tomography in acute ischemic stroke. World J Radiol 2010;2:354-7. doi: 10.4329/wjr.v2.i9.354.
Koo CK, Teasdale E, Muir KW. What constitutes a true hyperdense middle cerebral artery sign? Cerebrovasc Dis 2000;10:419-23. doi: 10.1159/000016101.
Chiang PL, Lin SY, Chen MH, Chen YS, Wang CK, Wu MC, et al. Deep learning-based automatic detection of ASPECTS in acute ischemic stroke: improving stroke assessment on CT scans. J Clin Med 2022;11:5159. doi: 10.3390/jcm11175159.
Austein F, Wodarg F, Jürgensen N, Huhndorf M, Meyne J, Lindner T, et al. Automated versus manual imaging assessment of early ischemic changes in acute stroke: comparison of two software packages and expert consensus. Eur Radiol 2019;29:6285-92. doi: 10.1007/s00330-019-06252-2.
Chen Z, Shi Z, Lu F, Li L, Li M, Wang S, et al. Validation of two automated ASPECTS software on non-contrast computed tomography scans of patients with acute ischemic stroke. Front Neurol 2023;14:1170955. doi: 10.3389/fneur.2023.1170955.
Neuhaus A, Seyedsaadat SM, Mihal D, Benson JC, Mark I, Kallmes DF, et al. Region-specific agreement in ASPECTS estimation between neuroradiologists and e-ASPECTS software. J Neurointerv Surg 2020;12:720-3. doi: 10.1136/neurintsurg-2019-015442.
Naganuma M, Tachibana A, Fuchigami T, Akahori S, Okumura S, Yi K, et al. Alberta Stroke Program Early CT Score calculation using the deep learning-based brain hemisphere comparison algorithm. J Stroke Cerebrovasc Dis 2021;30:105791. doi: 10.1016/j.jstrokecerebrovasdis.2021.105791.
Sawicki M, Safranow K, Wiska L, Pasek I, Gajdziel A, Gruszewski M, et al. Diagnostic value of artificial intelligence—based software in detection of large vessel occlusion in acute ischemic stroke. Appl Sci 2021;11:10017. https://doi.org/
3390/app112110017.
Wolff L, Berkhemer OA, van Es ACGM, van Zwam WH, Dippel DWJ, Majoie CBLM, et al. Validation of automated Alberta Stroke Program Early CT Score (ASPECTS) software for detection of early ischemic changes on non-contrast brain CT scans. Neuroradiology 2021;63:491-8. doi: 10.1007/s00234-020-02533-6.
Maegerlein C, Fischer J, Mönch S, Berndt M, Wunderlich S, Seifert CL, et al. Automated calculation of the Alberta Stroke Program Early CT Score: feasibility and reliability. Radiology 2019;291:141-8. doi: 10.1148/radiol.2019181228.
Temmen SE, Becks MJ, Schalekamp S, van Leeuwen KG, Meijer FJA. Duration and accuracy of automated stroke CT workflow with AI-supported intracranial large vessel occlusion detection. Sci Rep 2023;13:12551. doi: 10.1038/s41598-023-39831-x.
Löffler MT, Sollmann N, Mönch S, Friedrich B, Zimmer C, Baum T, et al. Improved reliability of automated ASPECTS evaluation using iterative model reconstruction from head CT scans. J Neuroimaging 2021;31:341-7. doi: 10.1111/jon.12810.
McTaggart RA, Jovin TG, Lansberg MG, Mlynash M, Jayaraman MV, Choudhri OA, et al. Alberta stroke program early computed tomographic scoring performance in a series of patients undergoing computed tomography and MRI: reader agreement, modality agreement, and outcome prediction. Stroke 2015;46:407-12. doi: 10.1161/STROKEAHA.114.006564.
Kuang H, Najm M, Chakraborty D, Maraj N, Sohn SI, Goyal M, et al. Automated ASPECTS on noncontrast CT scans in patients with acute ischemic stroke using machine learning. AJNR Am J Neuroradiol 2019;40:33-8. doi: 10.3174/ajnr.A5889.
Nagel S, Sinha D, Day D, Reith W, Chapot R, Papanagiotou P, et al. e-ASPECTS software is non-inferior to neuroradiologists in applying the ASPECT score to computed tomography scans of acute ischemic stroke patients. Int J Stroke 2017;12:615-22. doi: 10.1177/1747493016681020.
Guberina N, Dietrich U, Radbruch A, Goebel J, Deuschl C, Ringelstein A, et al. Detection of early infarction signs with machine learning-based diagnosis by means of the Alberta Stroke Program Early CT score (ASPECTS) in the clinical routine. Neuroradiology 2018;60:889-901. doi: 10.1007/s00234-018-2066-5.
Goebel J, Stenzel E, Guberina N, Wanke I, Koehrmann M, Kleinschnitz C, et al. Automated ASPECT rating: comparison between the Frontier ASPECT Score software and the Brainomix software. Neuroradiology 2018;60:1267-72. doi: 10.1007/s00234-018-2098-x.
Hoelter P, Muehlen I, Goelitz P, Beuscher V, Schwab S, Doerfler A. Automated ASPECT scoring in acute ischemic stroke: comparison of three software tools. Neuroradiology 2020;62:1231-8. doi: 10.1007/s00234-020-02439-3.
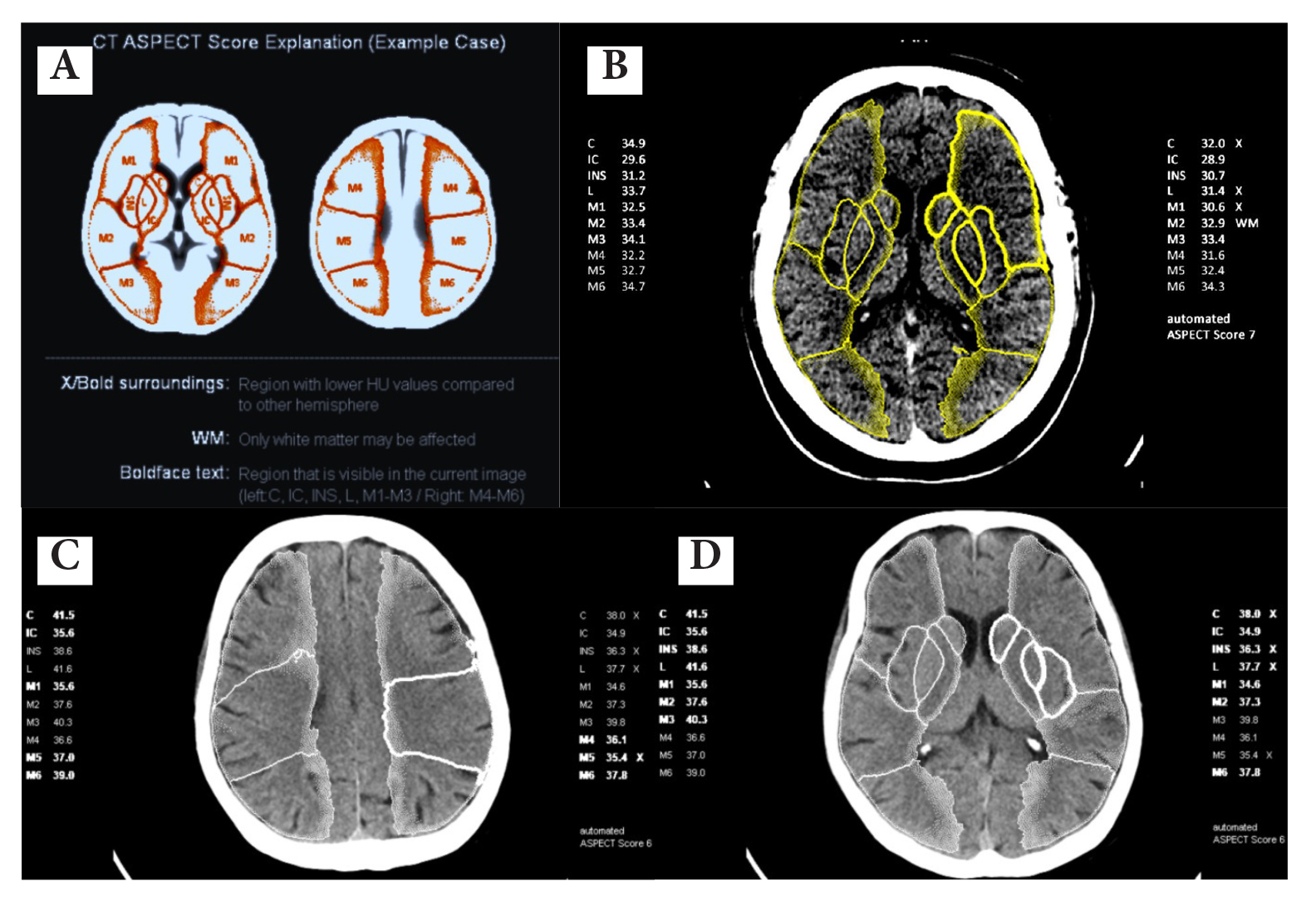
Downloads
Published
How to Cite
Issue
Section
License
Copyright (c) 2025 The ASEAN Journal of Radiology

This work is licensed under a Creative Commons Attribution-NonCommercial-NoDerivatives 4.0 International License.
Disclosure Forms and Copyright Agreements
All authors listed on the manuscript must complete both the electronic copyright agreement. (in the case of acceptance)